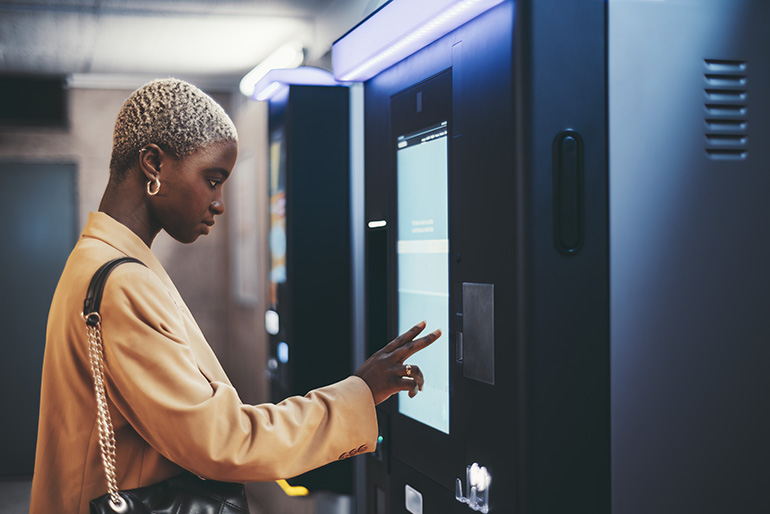
Artificial intelligence (AI) is ushering in a new era for the payments industry, offering transformative benefits in operational efficiency, security, and personalization that businesses can’t afford to overlook. When deployed strategically, AI has the potential to substantially reduce fraud rates, streamline existing workflows, and increase convenience for customers — all of which can help financial institutions, payment service providers (PSPs), and other organizations stay agile and meet rising customer expectations.
If there’s one thing that’s clear, it’s that businesses in the payments industry must strategically invest in AI technology to remain competitive. The integration of AI into payment systems not only promises to enhance service offerings but also provides critical insights through advanced data analytics. As AI reshapes the payments industry, businesses that leverage these innovations will be well-positioned to lead the market and evolve for the future.
AI: the latest and greatest in payments technology
Artificial intelligence is a broad field of computer science dedicated to creating systems capable of performing tasks that normally require human intelligence, such as learning, reasoning, problem-solving, perception, language comprehension, and dynamic interaction.
An umbrella term, AI consists of various technologies that mimic cognitive functions, making it a pivotal tool in modern computing and business operations:
Machine learning
Machine learning (ML) enables systems to learn and improve from experience without being explicitly programmed. ML algorithms achieve this by analyzing large datasets, identifying patterns, and making decisions based on the data. ML algorithms can engage in supervised, unsupervised, or reinforced learning.
Supervised learning involves training a model on a labeled dataset, where the desired output is already known. As its name implies, unsupervised learning is the inverse: A model is given data without explicit instructions on what to do with it, with the goal of identifying hidden patterns or intrinsic structures. Finally, reinforced learning involves training models through a system of rewards and penalties.
In the payments industry, machine learning is often used for fraud detection and prevention, analyzing vast quantities of transaction data in real-time to detect fraudulent activity. By continuously learning from new data, these systems improve their accuracy in flagging fraudulent transactions, which is crucial to protecting high-value transactions typical of business-to-business (B2B) environments.
Natural language processing
Natural language processing (NLP) is a form of ML that enables computers to understand, interpret, and respond to queries in human language. NLP has numerous applications, including sentiment analysis, language translation, and virtual assistants being some of the most common. Financial institutions and PSPs can use NLP-powered virtual agents to handle inquiries from business clients.
For commercial banks and account-to-account payment providers, NLP is invaluable for analyzing and interpreting contracts and compliance documents, ensuring that all transactions comply with relevant regulations and contractual obligations, which reduces legal risk.
Predictive analytics
Predictive analytics uses statistical techniques, ML, and data mining to analyze historical data and make predictions about future events. This powerful technology can help businesses in the payments industry forecast demand for their services so they can scale infrastructure accordingly and handle peak transactions volumes without disruptions. Financial institutions and PSPs can also leverage predictive analytics to forecast cash flow based on historical transaction data and business cycles, enabling them to better manage liquidity, plan for future expenses, and optimize working capital.
Neural networks
Neural networks, also known as deep learning, is another form of ML that aims to replicate the decision-making capabilities of the human brain through a system of connected nodes known as artificial neurons.
Neural networks can be a valuable asset for credit risk management and dynamic transaction routing. For the former, a neural network can assess the creditworthiness of businesses by analyzing various financial indicators and transaction histories, empowering financial institutions to make informed lending decisions. For the latter, neural networks can optimize transaction routing through different payment networks and schemes. This minimizes costs while maximizing speed, ensuring efficient processing for account-to-account payments.
Computer vision
Computer vision is the field of AI that trains computers to interpret and understand the visual world. By processing images and videos, AI systems can recognize objects, classify images, and detect anomalies. Businesses in the payments industry can use computer vision to automate the verification of documents such as invoices, purchase orders, and contracts, accelerating processing times and reducing the risk of error associated with manual verification.
A brief history of AI in payments
While AI might seem like more of a recent innovation, its history in the payments industry traces all the way back to the mid-1990s, with heavyweights such as Visa leveraging neural networks to support fraud detection and risk management. Though AI’s capabilities were a far cry then from what they are today, this early use of AI-enabled businesses to reduce financial losses and established a new standard for transaction security.
Moving into the early 2000s, AI’s role expanded to encompass more sophisticated risk management techniques, with financial institutions leveraging predictive analytics and neural networks to better assess credit risks and market trends, leading to more informed decision-making processes. Early adoption of this technology by leading institutions underscored growing reliance on AI to enhance operational efficiency and financial stability. The 2010s saw a significant leap with the introduction of chatbot technology and natural language processing, which enabled institutions to deliver more personalized client support and develop new service offerings.
Entering the 2020s, AI technology has rapidly matured, in no small part thanks to the emergence of large language models (LLMs) and generative AI. These innovations have broadened the scope of AI applications in the payments industry. One noteworthy example is JP Morgan’s Contract Intelligence (COIN) program, which uses machine learning to review legal documents and extract essential data points, dramatically reducing the time and cost associated with manual document review. According to one report, COIN was able to complete 360,000 hours of work in a matter of seconds — a monumental savings for the finance company.
Current attitudes toward AI in the payments industry reflect a strong belief in the technology’s transformative potential. A recent industry-wide survey revealed that 94% of senior payments professionals believe AI and ML are increasingly used to improve fraud detection. This widespread confidence and conviction is indicative of the significant advancements and proven success of AI in the payments industry over the years. Moreover, as AI continues to evolve and mature, future innovations will further enhance security, efficiency, and customer experience.
Looking ahead, the future of AI in banking and payments is poised for continued growth and innovation, and businesses can expect even more sophisticated tools for managing transactions, mitigating risks, and providing superior service. As AI becomes more ingrained in the financial ecosystem, it will drive significant improvements in how payments are processed and managed, ensuring the industry remains at the forefront of technological innovation.
9 applications for AI in payments
Integrating AI technology into existing workflows has the power to help financial institutions and PSPS enhance operations, deliver superior service, and maintain a competitive edge. Here are just a few AI use cases in the payments industry:
- Fraud detection: Leveraging advanced machine learning algorithms, AI systems can perform real-time analysis of transaction data to identify intricate patterns and anomalies indicative of fraudulent activity. These systems continuously learn and adapt to new fraud tactics, providing a dynamic defense against evolving threats. Financial institutions and PSPs can integrate these AI-driven solutions into their payment infrastructures to reduce false positives and enhance overall transaction security, maintaining customer trust and compliance with stringent regulatory requirements.
- Risk assessment: AI enhances risk assessment by integrating and analyzing diverse datasets, including financial statements, transactional histories, and real-time market data. Predictive analytics and neural networks provide a detailed risk profile for each business partner or transaction, enabling institutions to make more informed lending decisions and manage their risk exposure more effectively, optimizing portfolio performance and minimizing defaults.
- Know Your Customer (KYC): AI technologies, particularly those that utilize computer vision and machine learning, streamline the KYC process by automating complex corporate documents and identity verification. These systems can authenticate business licenses, financial reports, and other documentation, cross-referencing them with multiple data sources to ensure compliance with Anti-Money Laundering and Countering the Financing of Terrorism (AML/CFT) regulations.
- Transaction analysis: AI-driven tools provide deep insights into payment data, identifying trends and patterns that can reveal potential inefficiencies in transaction processing or risk. Advanced data analytics can optimize transaction workflows and detect early signs of payment delays or discrepancies. This proactive approach increases efficiency when managing large-scale account-to-account transactions, improving both operational resilience and client satisfaction.
- Client support: NLP-powered virtual agents and assistants can handle complex inquiries related to account management, payment processing, compliance issues, and more. These AI systems provide immediate, accurate responses based on existing policies and documentation, reducing the workload of support teams and enabling them to focus on high-value client interactions.
- Predictive analytics: Predictive analytics are vital to organizations in the payments industry, enabling them to forecast transaction volumes, detect potential fraud, and optimize cash flow management. By analyzing historical data and current market conditions, AI systems can provide actionable insights that improve strategic planning and increase operational efficiency, helping organizations anticipate and mitigate risk.
- Process Automation: When combined with robotic process automation (RPA), AI can automate highly repetitive tasks such as data entry, reconciliation, and compliance monitoring. By automating these tasks, financial institutions and PSPs can reduce human error, ensure greater accuracy when processing large volumes of transactions, and reallocate existing resources to more strategic activities.
- Intelligent Routing: Intelligent routing systems use machine learning algorithms to optimize payment processing for large-scale account-to-account transactions across multiple payment networks. By analyzing real-time and historical data, such as network fees, processing times, and currency conversion rates, intelligent routing systems can dynamically adjust routing paths to ensure fast and cost-effective transaction processing — an essential capability when managing high volumes of cross-border transactions.
- Liquidity Management: AI tools provide sophisticated liquidity management by offering real-time visibility into cash positions and forecasting future liquidity needs. Machine learning models analyze transactional data, market conditions, and other financial indicators to accurately predict cash flow requirements, enabling organizations to maintain optimal liquidity levels, reducing the risk of shortfalls or excess liquidity, and improving financial stability.
- Integration and Mapping: AI can greatly enhance the efficiency of data integration and mapping processes in the payments industry. Financial institutions often need to convert novel data formats into standard formats such as ISO 20022. Traditionally, this mapping process requires extensive manual coding and configuration; however, AI and ML can streamline this process by learning from incoming messages and predicting how different fields should be mapped to payment elements. This predictive capability can suggest the most suitable source-to-destination mappings for various message types, reducing the need for manual intervention and accelerating integration.
- Payments Intelligence and Insight Queries: Business intelligence tools and dashboards are essential for querying payment data and extracting valuable insights. AI, particularly through NLP capabilities supported by AI models such as ChatGPT, can reimagine how users interact with these tools. Instead of relying on traditional reporting and complex filters, users can leverage natural-language queries to obtain the information they need.
By leveraging AI for these and other applications, organizations in the payments industry can implement more robust security measures, dramatically increase operational efficiency, drive more informed decision-making, gain control over costs, and scale their systems over time to handle growing transaction volumes.
How AI is driving payments industry trends
The payments industry is experiencing a paradigm shift with the advent of real-time payments and the adoption of ISO 20022 messaging standards. These trends are simultaneously driving the need for AI and enhancing its abilities. This symbiotic relationship is shaping the future of payments, making them more efficient, secure, and sophisticated.
The industry-wide push for real-time payments necessitates that every aspect of the transaction process, from analytics to risk assessment, operates in real-time — and AI is uniquely positioned to meet this demand. As payments become instantaneous, financial institutions, PSPs, and other organizations must be able to deliver immediate fraud detection, transaction monitoring, and compliance checks. AI-based solutions enable them to rise to this demand, analyzing vast quantities of data in milliseconds and identifying anomalies and potential threats faster than traditional systems.
AI’s ability to perform real-time translation and conversation is instrumental in cross-border transactions, where language barriers can slow down the process. AI can instantly translate transaction details and communications, ensuring seamless transactions and reducing delays. Similarly, image recognition technology can quickly verify the authenticity of documents and payment proofs, further accelerating transaction times.
ISO 20022 has also revolutionized the payments industry by introducing a flood of new, more granular data. This data is both structured and unstructured and encompasses everything from standardized transaction fields to freeform supply chain information such as invoices, purchase orders, PDFs, and images of bills. The structured data enables systems to understand the meaning of each field, while the unstructured data provides a rich source of information that requires sophisticated processing.
AI technology is essential for handling this influx of data. ML algorithms can parse and interpret unstructured data, extracting valuable insights and ensuring that no critical information is overlooked. Moreover, the vast amounts of data ISO 20022 makes readily available provide AI systems with the “fuel” they need to learn and improve. The more data AI systems process, the more accurate and efficient they become. This continuous learning loop enhances AI’s ability to deliver real-time analytics, predictive risk assessments, and personalized payment experiences.
As AI capabilities mature, they will play an increasingly vital role in the payments ecosystem, optimizing transaction routing, providing real-time insights into transaction patterns, and enabling businesses to make data-driven decisions on the fly. Amidst these conditions, embracing AI isn’t just an option but a necessity for businesses looking to thrive in this rapidly changing landscape.
Key things to watch out for when using AI
For all its benefits, AI also introduces several challenges that organizations must be mindful of when utilizing this technology:
Data privacy concerns
One of the foremost challenges in implementing AI is ensuring data privacy. AI and ML algorithms need to be trained on large datasets to develop expertise. Although the aggregate universe of payments data is vast, much of it sits behind firewalls and is subject to stringent privacy regulations, such as the General Data Protection Regulation (GDPR) and the California Consumer Privacy Act (CCPA). This limits the accessibility of data necessary for training AI models effectively.
To overcome this challenge, financial institutions can adopt innovative approaches such as federated learning, where AI models are trained on decentralized devices holding local data samples without sharing them. This method supports robust AI model training while keeping data within local environments, thus maintaining privacy and regulatory compliance.
Additionally, techniques such as data anonymization, pseudonymization, and synthetic data generation can provide AI systems with valuable training data without compromising actual customer information. Collaborative data-sharing agreements with other organizations — under strict privacy guidelines, of course — can also facilitate comprehensive datasets for AI training. Finally, implementing strong data governance frameworks and adopting privacy-preserving AI techniques such as homomorphic encryption further maintain data privacy throughout the training process.
Need for human oversight
Despite its advanced capabilities, AI still requires human oversight to ensure ethical decision-making and accountability. AI systems can sometimes produce biased or incorrect results due to flawed algorithms or biased training data. Businesses can address these biases by adopting a human-in-the-loop approach, wherein human experts regularly review AI outputs and intervene when necessary. Establishing clear guidelines and protocols for AI oversight can maintain the balance between automation and human judgment, ensuring AI decisions align with organizational values and regulatory requirements.
Implementation costs
The initial investment AI systems require can be substantial, covering costs for technology acquisition, system integration, personnel training, and more. Adopting a phased approach to AI deployment, starting with pilot projects that demonstrate a clear return on investment, can help organizations leverage AI while controlling costs. Utilizing cloud-based AI services can also reduce upfront costs, as they offer scalable solutions without the need for significant infrastructure investment, lowering the barrier to implementation.
Ethical considerations
The use of AI in the payments industry raises important ethical considerations, including transparency, fairness, and accountability. AI systems must be designed and deployed in such a way that they do not perpetuate biases or discriminate against any group.
To navigate these ethical challenges, financial institutions and PSPs should build ethical AI frameworks, ensuring that AI development and deployment adhere to principles of fairness and transparency. Regular assessments of AI systems can further identify and address ethical issues, fostering trust among stakeholders and clients.
Fraud sophistication
As AI technology advances, so, too, do fraudsters’ methods, with many using AI-driven schemes to avoid detection by traditional systems. To stay one step ahead of these evolving threats, organizations in the payments industry must implement adaptive AI systems capable of learning and evolving in real-time. It’s essential that organizations continuously monitor and update their AI models to detect and counteract new fraud techniques. Collaborating with industry peers and participating in information-sharing networks can also improve organizations’ ability to effectively respond to emerging fraud trends.
Challenges with structured data
AI and ML applications face unique challenges with core account-to-account payment processing due to the structured nature of payments data. ISO 20022 messages are highly structured and self-documenting, which often makes the use of complex AI and ML models unnecessary. Generative AI, in particular, struggles with non-narrative data formats such as this, as it is primarily designed to handle narrative text-based data.
For applications involving structured payments data, simpler and more efficient methods can generate the required insights without the overhead of AI. However, there are still valuable adjacent use cases for this technology, such as enhancing data integration and mapping processes. By focusing AI efforts on these areas, financial institutions can optimize operations without overcomplicating their core payment processing systems.
Performance concerns
Real-time payments must meet stringent service level agreements (SLAs); incorporating AI into core processing workflows can complicate this. AI algorithms, particularly those used in real-time fraud detection and compliance checks, can introduce latency and require significant processing power, hindering the ability to process transactions quickly and potentially compromising SLAs.
To maintain high performance when integrating AI, institutions should carefully evaluate use cases for AI and ML within their core systems. AI and ML can be highly effective in peripheral systems, such as fraud detection and anti-money laundering, where real-time processing is crucial without impacting core transaction processing speeds.
What does the future hold for AI in payments?
AI is fast maturing, with new applications for organizations in the payments industry emerging each day. Here are some key trends to watch:
- A unified front against fraud: AI-driven fraud detection algorithms will continue to mature, leveraging deep learning and advanced pattern recognition techniques to not only identify fraudulent activity but also predict and preempt it. Financial institutions and PSPs will increasingly work in tandem with regulatory bodies to share intelligence and develop standardized protocols for combating fraud, creating a united front against cyber threats and ensuring robust security measures across the payments industry.
- Data-driven decision-making: Organizations will harness AI to perform real-time risk evaluations, integrating a variety of data points to make more informed lending and transaction decisions. Real-time analytics will also power dynamic pricing strategies, enabling businesses to adjust their offerings based on current market conditions and client behavior. Personalized offers and recommendations informed by AI insights will further enhance client engagement and satisfaction, providing tailored financial products and services that meet corporate clients’ needs.
- Emerging payment models: The adoption of new payment models, particularly those based on blockchain technology, will continue to have a significant impact on the payments industry. Blockchain’s decentralized ledger provides a secure, transparent, and efficient framework for processing transactions, reducing costs and the need for intermediaries. Digital currencies, including central bank digital currencies (CBDCs), will become more prevalent, offering a stable and regulated alternative to traditional currencies. AI will be integral in managing these new payment models, supporting their efficiency, security, and integration with existing financial infrastructure.
- Increased industry collaboration: Looking ahead, we can expect to see increased collaboration between traditional financial institutions and fintech companies, combining the stability and regulatory expertise of incumbent banks with the agility and innovation of startups. These partnerships will address various payment ecosystem challenges, from accelerating straight-through processing to developing new financial products. Joint ventures will drive innovation, enabling both fintech and traditional institutions to lean on their strengths and create comprehensive solutions that cater to the evolving needs of the market.
Discover how Volante is supporting AI in payments
At Volante, we’re dedicated to advancing the capabilities of financial institutions and PSPs through powerful AI integration. By helping these institutions modernize their payment processing infrastructures, we ensure that they’re able to leverage AI to its fullest potential, meeting the growing demand for real-time, data-rich operations.
We offer a comprehensive suite of solutions designed to seamlessly incorporate AI into your existing operations, including:
- Payments as a Service: Our cloud-native platform ensures rapid deployment of AI-driven functionalities, enhancing real-time payment processing and customer experience.
- Real-Time Payments: Our real-time payments solution supports 24×7 instant payment processing, integrating AI to enhance fraud detection and compliance.
- ISO 20022 Service: Build ISO 20022-based services using low-code development and AI tools to leverage structured data for better analytics and decision-making with our ISO 20022 service.
- APIs: Embed the full power of Volante’s payments processing technology into existing or new AI-enabled applications with over 700 smart APIs.
To learn more about these and Volante’s other offerings, contact our team of payment experts today.